Federated Learning For Privacy-Preserving
There has been tremendous growth in the field of AI and machine learning. The developments across these fields have resulted in a considerable increase in other FinTech fields. Cyber security has been described as an essential part of the developments associated with technology. Increased cyber security ensures that
people remain protected, and that data remains safe. New methods have been integrated into developing AI that achieves cyber security. The data analysis capabilities of AI and its cyber security functions have ensured that privacy has increased significantly. The ethical concept associated with data privacy has also been advocated across most FinTech regulations. These concepts and considerations have all been engaged with the need to achieve the required ethical requirements. The concept of federated learning is a recently developed measure that achieves the above mentioned concept. It ensured the development of AI and machine learning while keeping privacy in data analysis. The research paper effectively describes the issue of federated learning for confidentiality. It describes the overall process associated with its
development and some of the contributions it has achieved. The widespread application of federated learning in FinTech is showcased, and why federated learning is essential for overall growth in FinTech.
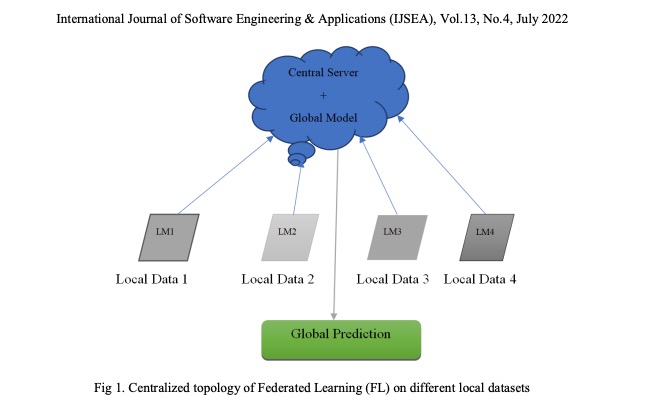
By definition, “Federated learning is a machine learning setting where multiple entities (clients) collaborate in solving a machine learning problem, under the coordination of a central server or service provider. Each client’s raw data is stored locally and not exchanged or transferred; instead, focused updates for immediate aggregation are used to achieve the learning objective.As described above, the primary use of federated learning was to promote privacy for data scientists and researchers. Centralized learning and data collection have been described as a potential risk for users’ data [9]. Organizations significantly benefit from increased privacy which ensures they have avoided legal issues. The risks associated with organizations being sued have increased the need for using the decentralized model. The expanding community associated with
federated learning indicates that it achieves the required level of privacy that organizations and scientists require