Artificial Intelligence in the Era of Quantum Computing: A Review
Quantum computing and artificial intelligence (AI) are both transformational technologies. Quantum computing can provide a computation boost to AI, enabling it to tackle more complex problems in many fields in business and science. Although AI produces functional applications with classical computers, it is limited by the computational capabilities of classical computers. Quantum-enhanced machine learning refers to quantum algorithms that solve tasks in machine learning, thereby improving and often expediting classical machine learning techniques. Quantum machine learning is the integration of quantum algorithms within machine learning programs. The most common use of the term refers to machine learning algorithms for the analysis of classical data executed on a quantum computer 2. Quantum AI can improve outcomes in ML by improving upon tried and tested ML techniques. This will lead to improved prediction rates and pattern recognition but can also be applied for unsupervised learning, clustering, and anomaly detection. The quantum computing solutions can help AI and ML operations by providing a computation boost to tackle more complex problems and improve outcomes in ML by improving upon tried and tested ML techniques.
Quantum machine learning is the integration of quantum algorithms within machine learning programs. It can be used to analyze classical data, quantum states, or both on a quantum computer or a classical device. Quantum-enhanced machine learning refers to quantum algorithms that solve tasks in machine learning, thereby improving and often expediting classical machine learning techniques. Quantum machine learning utilizes qubits and quantum operations or specialized quantum systems to improve computational speed and data storage done by algorithms in a program. Hybrid methods that involve both classical and quantum processing can also be used, where computationally difficult subroutines are outsourced to a quantum device 1. These routines can be more complex in nature and executed faster on a quantum computer.
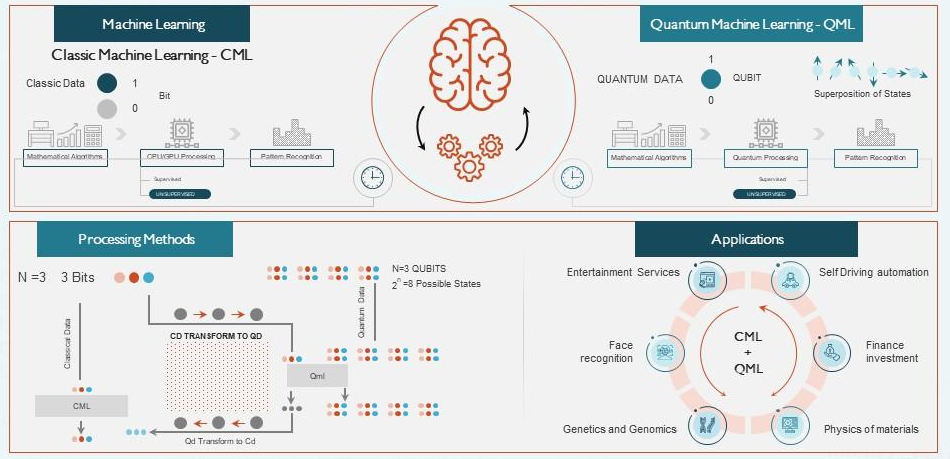
Quantum machine learning is a subfield of machine learning that uses quantum computing to perform certain tasks more efficiently than classical computers. It utilizes qubits and quantum operations or specialized quantum systems to improve computational speed and data storage done by algorithms in a program. Hybrid methods that involve both classical and quantum processing can also be used, where computationally difficult subroutines are outsourced to a quantum device.
Benefits:
Quantum machine learning is a subfield of machine learning that uses quantum computing to perform certain tasks more efficiently than classical computers. Here are some benefits of quantum machine learning:
Faster processing: Quantum computers can process large amounts of data exponentially faster than classical computers, which can lead to faster training of machine learning models.
Improved accuracy: Quantum machine learning can improve the accuracy of machine learning models by reducing the number of iterations required to reach a solution.
Better scalability: Quantum machine learning can handle larger datasets than classical machine learning algorithms, which can lead to better scalability.
New applications: Quantum machine learning can enable new applications in fields such as finance, healthcare, and logistics, where classical machine learning algorithms are limited by computational power.